“Before we wake up and find that year 2024 looks like the book “1984”, let’s figure out what kind of world we want to create on technology”
March 23, 2016, Microsoft launches on Twitter its cutting-edge AI solution named- Tay However, it took less than a day for Tay, this playful conversational AI chatbot, to become crooked. All because of a crash course on racism and bias it underwent from fellow Twitter users.
For all these reasons, Microsoft had to pull the plug on Tay and scrap the project completely. October 2018 Amazon scrapped its much-anticipated AI Recruitment tool it had been building since 2014 because it didn’t like women and was biased against them. Primarily because there was an inherent bias in the training data used in favour of men from the CV’s submitted over a 10-year period. As a result, the AI solution rated women resumes consistently lower than men. The project was secretly scrapped by Amazon. And there are several such instances in the AI world. All these examples bring up a very pertinent question about AI adoption i.e. do we humans completely understand the ramifications of AI adoption? And the voice that echoes in unison in all our discussions with our clients is a stupendous No. This really means that there is a burning need for organizations to embed ethics in their AI strategies. And in this article, we share our perspective on how to do that.
Some of the ethical questions that surface with AI adoption strategy are-
- AI Singularity: Will we end up creating something that is more intelligent than us?
Can we ensure that we will be in complete control or will we end up losing control over AI? - Job Apocalypse: Will AI ruthlessly take away livelihoods of people?
- Lack of Transparency: With each new release of sophisticated Deep Learning architecture, a question that begs an answer is- Are we building something so sophisticated that its unexplainable even for us?
- Inclusion & Diversity: Are we building something which has an inherent bias and is not inclusive?
Eg- Uber’s Facial recognition AI solution blocked transgenders drivers from the system as it wasn’t trained on LGBT data, in turn costing them their jobs.
These questions rightly bring in the concept of building Responsible AI
What do we mean by Responsible AI?
Microsoft has laid out its internal guiding principles which summarize Responsible AI under the following six heads
- Fairness: AI solution that doesn’t have any algorithmic bias and treats everyone fairly
- Reliability and Safety: Human machine trust stays intact all the time
- Privacy and security: the AI system should know where to draw the line to not invade consumers privacy and security
- Inclusiveness: AI should be empowering and not overpowering
- Transparency & Explainability: We should be able to explain what we have built
- Accountability: Companies should be accountable for the actions of their AI system
Therefore, designing trustworthy AI solutions that reflect human ethical principles such as the ones above is what Responsible AI means. The above is by no means exhaustive set of principles but is one perspective on steps towards Responsible AI. So, what should be done to ensure that our AI doesn’t see the doomsday? What are some ways to embed Responsible AI in our corporate ethos?
We answer this question at following two tiers
Global
Carving out globally accepted set of principles for Ethics in AI are paramount. Globally at least 84 public-private initiatives are underway to describe high level principles on ethical AI. We need to have a convergence across these initiatives at a global level and compliance guidelines akin to GDPR with heavy downsides for an organization for non-compliance.
Organizational
To start with, we feel that there is a dire need for Corporate Governance frameworks to position AI as an alliance of a machine’s IQ with a human’s EQ rather than it being an autonomous solution. Thereafter formulate initiatives around:
- Cultural awareness within the organization towards Ethical AI & its framework
- Guidelines around retraining & reskilling people about to be impacted by the implementation of AI
- Ensuring legal & regulatory compliance as a core component of AI scenario development
- Algorithm Auditing- Ensure complete authority for solution owners to work around the AI principles and audit the compliance at any given time using algorithm auditing in lab environments
With these meaningful steps, organizations can make meaningful strides towards building trustworthy AI.
If you’ve enjoyed reading this article, hit like, share and comment. We would love to hear your thoughts on building Responsible AI. Do you feel the business need for Responsible AI? Have you and/or your organization built a roadmap for the same?
Disclaimer: Through these guidelines, we don’t anticipate creation of a utopian AI world which is completely flawless, but this is our (and other like-minded organizations’) proposition to ensure that we don’t end up in a dystopian world and that we continue to work towards a better future for all of us Acknowledgement: Microsoft, Accenture, INSEAD, University of Oxford References: https://papers.ssrn.com/sol3/papers.cfm?abstract_id=3391293
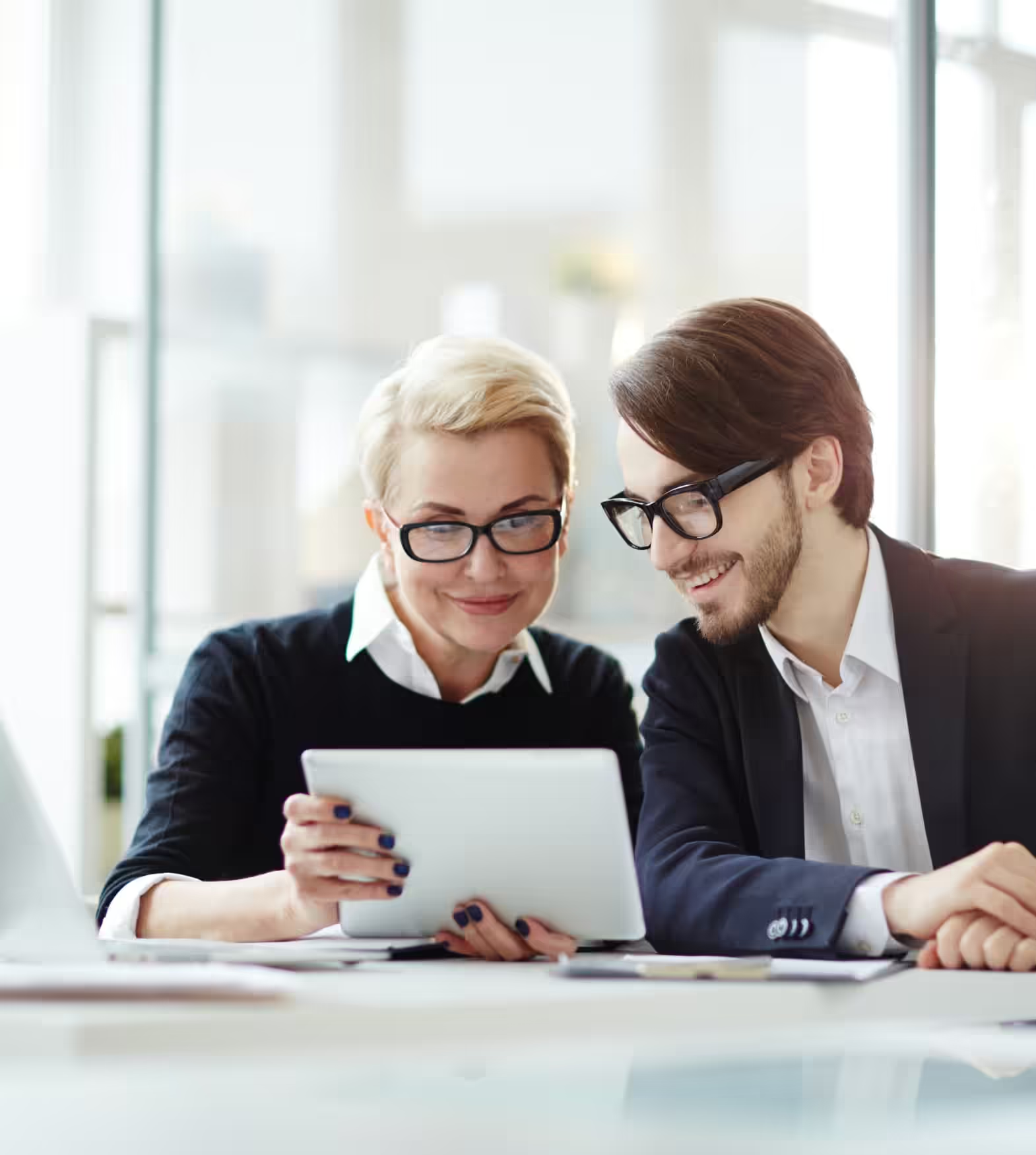
Need Help?
Reach out to learn more about our team and the kinds of tailored solutions we can offer your organization.
Get in Touch